OR/15/001 Validation results
Jordan, J J, Grebby, S, Dijkstra, T, Dashwood, C and Cigna, F. 2015. Risk information services for Disaster Risk Management (DRM) in the Caribbean. British Geological Survey Internal Report, OR/15/001. |
Quality checking
Service 1: Land use/land cover mapping
Initial quality checks comprised evaluating whether the land use/land cover products satisfied the minimum requirements outlined in the SOW. Regarding spatial coverage, the minimum coverage of 80% of the AOIs was achieved by using the RapidEye imagery and existing land use/land cover maps to infill areas significantly obscured by cloud and shadowing in the Pleiades imagery. As a result, the land use/land cover maps cover 100% of the AOI.
Water features (e.g. lakes, ponds, rivers, streams) and the basic road network could only be delineated on the pan-sharpened Pleiades imagery. Therefore, the completeness of the water features and road vector layers was dependent on cloud and shadow in the Pleiades imagery, as well as the areal coverage of any existing relevant information. Although mapping of such features was hindered by cloud and shadow — resulting in a somewhat fragmented dataset in some areas — the vector layers satisfy the minimum coverage requirements 80%. The satellite imagery used for mapping in this service was required in the last 3 years.
The thematic accuracy of the land use/land cover maps was determined using the conventional remote sensing approach of deriving confusion matrices (Congalton, 1991[1]The confusion matrices for the three AOIs are shown in Tables 8–10. In all cases, the overall accuracies of the maps achieve the target accuracy of 80–90%, with accuracies for St. Lucia, Grenada, and St. Vincent and the Grenadines of 84.9%, 84.8% and 80.8%, respectively. These accuracies were further confirmed through point-location field observations made in St. Lucia and Grenada during validation of the landslide inventories produced in Service 2.
The confusion matrices provide a useful insight into the confusion between classes that affect the land use/land cover maps. From Tables 8–10, it is clear that most of the confusion occurs between different types of vegetation, particularly forests. This can be expected because many of the classes are inherently similar. In St. Lucia, for example, 'Lowland forest' and 'Evergreen forest' both contain Evergreen trees, thus accounting for the observed confusion. Likewise, in Grenada, similarities and overlap between results in some degree of confusion between classes such as 'Semi-deciduous forest', 'Drought Deciduous open woodland', 'Deciduous, coastal Evergreen and mixed forest or shrubland' and 'Evergreen and seasonal Evergreen forest'. Enhanced discrimination between these classes would largely depend on acquiring satellite imagery to capture seasonal variations (i.e. leaf-on and leaf-off conditions). To a lesser degree, confusion between 'Bare ground', 'buildings' and 'Roads and other built-up surfaces' exists in all three maps. Again, this is not entirely unexpected because of colour similarities between these land use/land cover types. For example, bare rock or soil is a similar colour to buildings with reddish roofs, in the imagery, whereas grey buildings appear similar to surfaces made of concrete or asphalt roads. Similarly, some non-metalled or dusty roads also appear similar to bare rock or soil. Enhanced discrimination of these land use/land cover types would require more spectral information than that captured by the 4 multispectral bands of the Pleiades sensor.
Nevertheless, observations both in the field and imagery confirm that this type of confusion has been reduced considerably in the new land use/land cover maps in comparison to the existing maps (Figure 45). However, it is worth noting that a relatively minor degree of confusion does still persist around the coastline, marking the transition from land to the sea. This is most likely the result of the colour similarities between roads, light grey buildings and a sub-pixel mixing of sand/rock and the wake caused by breaking waves on the coast.
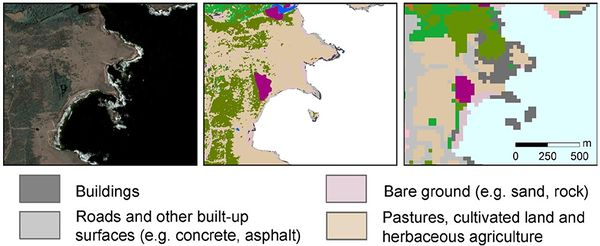
Validation data |
User’s accuracy (%) | |||||||||||||||
Mapped as | Water | Pasture | Semi‐decid | Lowland | Buildings | Roads | Bare ground | Elfin | Evergreen forest | Golf course | Mangrove | Wetland | Quarry | Woody agric | ||
1249 |
0 |
0 |
0 |
19 |
83 |
0 |
0 |
8 |
0 |
0 |
0 |
0 |
0 |
91.9 | ||
Pasture | 0 |
740 |
96 |
149 |
25 |
6 |
69 |
444 |
81 |
0 |
0 |
0 |
0 |
77 |
79.8 | |
Semi‐decid | 0 |
120 | 6003 |
942 |
0 |
0 |
0 |
0 |
79 |
0 |
138 |
0 |
0 |
527 |
76.9 | |
Lowland | 0 |
0 |
112 |
3886 |
0 |
9 |
2 |
0 |
353 |
0 |
25 |
0 |
0 |
570 |
78.4 | |
Buildings | 0 |
110 |
0 |
1 |
845 |
74 |
45 |
0 |
0 |
0 |
0 |
0 |
0 |
0 |
78.6 | |
Roads | 0 |
0 |
0 |
0 |
180 |
1501 |
151 |
0 |
0 |
0 |
0 |
0 |
0 |
0 |
81.9 | |
Bare ground | 0 |
64 |
0 |
0 |
96 |
17 |
292 |
0 |
0 |
0 |
0 |
0 |
0 |
0 |
62.3 | |
Elfin | 0 |
0 |
0 |
0 |
0 |
0 |
0 |
1703 |
179 |
0 |
0 |
0 |
0 |
0 |
90.5 | |
Evergreen | 0 |
0 |
0 |
0 |
0 |
0 |
0 |
232 |
2901 |
0 |
0 |
0 |
0 |
0 |
92.6 | |
Golf | 0 |
0 |
0 |
0 |
0 |
0 |
0 |
0 |
0 |
1048 |
0 |
0 |
0 |
0 |
100.0 | |
Mangrove | 0 |
0 |
0 |
0 |
0 |
0 |
0 |
0 |
0 |
0 |
2712 |
0 |
0 |
0 |
100.0 | |
Wetland | 0 |
0 |
0 |
0 |
0 |
0 |
0 |
0 |
0 |
0 |
0 |
590 |
0 |
0 |
100.0 | |
Quarry | 0 |
0 |
0 |
0 |
0 |
0 |
0 |
0 |
0 |
0 |
0 |
0 |
619 |
0 |
100.0 | |
Woody agric | 0 |
0 |
0 |
0 |
0 |
0 |
0 |
0 |
0 |
0 |
0 |
0 |
0 |
1583 |
100.0 | |
Producer’s accuracy (%) | 100.0 |
92.7 |
96.6 |
78.1 |
72.5 |
88.8 |
52.2 |
71.6 |
80.6 |
100.0 |
94.3 |
100.0 |
100.0 |
57.4 |
||
Overall accuracy (%) | 84.9% (K = 0.83) |
Validation data |
User’s accuracy (%) | ||||||||||||||||
Mapped as | Nutmeg | Pasture | Drought | Decid | Semi‐decid | Evergreen | Elfin | Bare ground | Buildings | Roads | Golf course | Wetland | Mangrove | Quarry | Water | ||
Nutmeg | 2047 |
393 |
13 |
168 |
163 |
262 |
0 |
1 |
28 |
0 |
0 |
0 |
21 |
1 |
0 |
66.1 | |
Pasture | 52 |
2126 |
0 |
224 |
14 |
0 |
0 |
120 |
52 |
3 |
0 |
0 |
2 |
0 |
0 |
82.0 | |
Drought | 0 |
0 |
622 |
7 |
3 |
0 |
0 |
0 |
0 |
0 |
0 |
0 |
13 |
0 |
0 |
96.4 | |
Decid | 95 |
365 |
175 |
5013 |
395 |
4 |
0 |
6 |
25 |
1 |
0 |
0 |
44 |
1 |
0 |
82.0 | |
Semi‐decid | 381 |
41 |
4 |
72 |
1733 |
185 |
0 |
0 |
1 |
0 |
0 |
0 |
4 |
0 |
0 |
71.6 | |
Evergreen | 471 |
69 |
13 |
235 |
212 |
5283 |
0 |
0 |
0 |
0 |
0 |
0 |
12 |
0 |
0 |
83.9 | |
Elfin | 101 |
54 |
0 |
0 |
0 |
73 |
3910 |
0 |
0 |
0 |
0 |
0 |
0 |
0 |
0 |
94.5 | |
Bare ground | 0 |
1 |
0 |
9 |
0 |
0 |
0 |
1073 |
9 |
35 |
0 |
0 |
0 |
0 |
0 |
95.2 | |
Buildings | 0 |
0 |
0 |
11 |
0 |
1 |
0 |
168 |
1103 |
56 |
0 |
0 |
0 |
0 |
0 |
83.1 | |
Roads | 0 |
0 |
0 |
0 |
0 |
0 |
0 |
99 |
133 |
1123 |
0 |
0 |
0 |
0 |
0 |
82.1 | |
Golf course | 0 |
0 |
0 |
0 |
0 |
0 |
0 |
0 |
0 |
0 |
296 |
0 |
0 |
0 |
0 |
100.0 | |
Wetland | 0 |
0 |
0 |
0 |
0 |
0 |
0 |
0 |
0 |
0 |
0 |
860 |
0 |
0 |
0 |
100.0 | |
Mangrove | 0 |
0 |
0 |
0 |
0 |
0 |
0 |
0 |
0 |
0 |
0 |
0 |
1699 |
0 |
0 |
100.0 | |
Quarry | 0 |
0 |
0 |
0 |
0 |
0 |
0 |
0 |
0 |
0 |
0 |
0 |
0 |
437 |
0 |
100.0 | |
Water | 0 |
0 |
0 |
0 |
0 |
0 |
0 |
0 |
7 |
3 |
0 |
0 |
9 |
0 |
1305 |
98.6 | |
Producer’s accuracy (%) | 65.0 |
69.7 |
75.2 |
87.3 |
68.8 |
91.0 |
100.0 |
73.1 |
81.2 |
92.0 |
100.0 |
100.0 |
94.2 |
99.5 |
100.0 |
||
Overall accuracy (%) | 84.8% (K = 0.83) |
Validation data |
User’s accuracy (%) | ||||||||||||||||
Mapped as | Evergreen | Seasonal | Semi‐decid | Pasture | Bare ground | Roads | Buildings | Drought | Water | Elfin | Woody agric | Montane | Blue Maho | Golf course | Mangrove | ||
Evergreen | 2882 |
1088 |
1419 |
5 |
2 |
9 |
15 |
86 |
0 |
283 |
0 |
0 |
0 |
0 |
0 |
49.8 | |
Seasonal | 11 |
867 |
37 |
0 |
0 |
0 |
0 |
3 |
0 |
2 |
0 |
0 |
0 |
0 |
0 |
94.2 | |
Semi‐decid | 928 |
273 |
2764 |
315 |
0 |
0 |
0 |
1239 |
0 |
25 |
0 |
0 |
0 |
0 |
0 |
49.9 | |
Pasture | 29 |
0 |
25 |
7023 |
866 |
26 |
85 |
482 |
0 |
1271 |
259 |
0 |
0 |
0 |
0 |
69.8 | |
Bare ground | 0 |
0 |
1 |
5 |
3450 |
8 |
15 |
4 |
0 |
0 |
0 |
14 |
0 |
0 |
0 |
98.7 | |
Roads | 0 |
0 |
0 |
2 |
258 |
1501 |
169 |
0 |
0 |
0 |
0 |
0 |
0 |
0 |
0 |
77.8 | |
Buildings | 0 |
0 |
0 |
12 |
52 |
64 |
1693 |
0 |
0 |
0 |
0 |
0 |
0 |
0 |
0 |
93.0 | |
Drought | 42 |
82 |
436 |
85 |
99 |
10 |
12 |
10954 |
0 |
540 |
0 |
0 |
0 |
0 |
0 |
89.3 | |
Water | 0 |
0 |
0 |
21 |
1 |
0 |
0 |
0 |
2131 |
0 |
0 |
0 |
0 |
0 |
0 |
99.0 | |
Elfin | 0 |
0 |
0 |
4 |
0 |
0 |
0 |
0 |
0 |
4992 |
0 |
234 |
9 |
0 |
0 |
95.3 | |
Woody agric | 0 |
0 |
226 |
0 |
0 |
0 |
0 |
0 |
0 |
0 |
4351 |
0 |
0 |
0 |
0 |
95.1 | |
Montane | 0 |
0 |
0 |
0 |
0 |
0 |
0 |
0 |
0 |
40 |
0 |
506 |
0 |
0 |
0 |
92.7 | |
Blue Maho | 0 |
0 |
0 |
0 |
0 |
0 |
0 |
0 |
0 |
0 |
0 |
0 |
484 |
0 |
0 |
100.0 | |
Golf course | 0 |
0 |
0 |
0 |
0 |
0 |
0 |
0 |
0 |
0 |
0 |
0 |
0 |
463 |
0 |
100.0 | |
Mangrove | 0 |
0 |
0 |
0 |
0 |
0 |
0 |
0 |
0 |
0 |
0 |
0 |
0 |
0 |
3224 |
100.0 | |
Producer’s accuracy (%) | 74.0 |
37.5 |
56.3 |
94.0 |
73.0 |
92.8 |
85.1 |
85.8 |
100.0 |
69.8 |
94.4 |
67.1 |
98.2 |
100.0 |
100.0 |
||
Overall accuracy (%) | 80.8% (K = 0.78) |
Service 2: Hazard mapping to support landslide risk assessment
Landslide inventories
The identification of landslide polygons was quality checked by two operators independently mapping two test areas in St Lucia. The results were compared and this led to fine-tuning of interpretation of indicators and establishment of observational guidance (landuse, bare soil signatures, use of elevation models, minimum landslide size, and use of landslide clusters). The final products were again cross-checked by two engineering geologists. Following the establishment of the multi-temporal inventory the results were evaluated against the 2010 landslide inventory. It was observed that there were some differences between the multi- temporal inventory and the 2010 inventory and these were all carefully evaluated. Generally, these differences were the result of an over-emphasis on ‘bare-earth’ mapping in the 2010 inventory. Additional differences were observed with the more conservative outlines of landslide areas in the multi-temporal inventory as long runouts were not mapped.
The completeness of landslide inventory is a function of the capability of the mode of recording of all the events over a period of time. Clearly, there are several issues therefore that influence completeness. In the case of this project these include, aspects of image resolution, of scale, and of the length of time over which observations are made. Visibility (absence of cloud cover, canopy overhangs, shadow effects, etc.) further influences the completeness of the landslide inventory.
To achieve an insight into the completeness of the landslide inventory of St Lucia, the complete multi-temporal dataset has been analysed. The extent of an individual landslide throughout the multi-temporal dataset is not constant. Deviations from this extent will occur in different years. This can occur through, for example, re-establishment of a vegetation cover leading to increasingly smaller areas remaining ‘active’. But it is also possible that slides extent, either downslope as a consequence of continuing displacement of a landslide mass, or upslope and laterally through retrogressive failure involving increasing large amounts of slope. For this exercise, landslide polygons offer an outline representing the maximum extent of an event throughout the time series. It is therefore unrealistic to construct area frequency diagrams for each year.
The analysis of the full dataset (incorporating the dataset mapped at 1:10k) resulted in a cumulative frequency-area distribution shown in Figure 46, following an approach outlined by Guzzetti 2005[2] and Malamud et al 2004[3] (see also Hurst et al. 2014[4]). It is interesting that spill-overs (i.e where the largest number of events are found of a particular size) depend on the nature of the survey. For quick reconnaissance surveys this spill-over lies at a characteristic size of about 104 to 105m2 (i.e. landslide dimensions of approximately 100x100m to 300x300m). For more detailed geomorphological surveys greater detail can be captured and this results in a trend with a spill-over at about 103m2 (indicative dimensions of about 30x30m) which is similar to what was found in our survey.
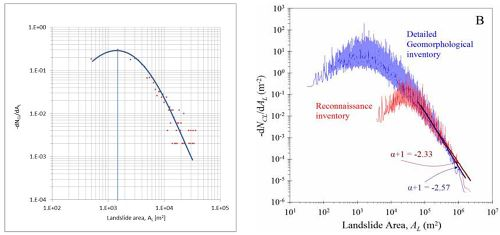
Even though from the above it appears that the established inventory provides a degree of confidence that it forms a fair representation of events there are known omissions from the database.
Minimum dimensions in the database are approximately 200 m2 and many smaller events are known to have occurred. Very small (<5 m) and obscured (in the shade, on steep slopes, below overhanging vegetation) landslides are difficult to capture but are known to exist in substantial numbers. These small events have a significant impact on lives and livelihoods and recording these through different means will be important to add to the database. It is possible to interrogate the recent Pleiades images (at resolution of 0.5m) at a greater level of detail and it is therefore advantageous to carry out future assessments at scales of 1:5 000 or better. The case study of Chateau Belair (discussed in the Executive summary) highlights the restrictions in using satellite images for interpretation. Subtle changes in topography can be interpreted by skilled operators as signatures of landslides in a complex terrain. At high resolutions (1:10k and better) this can lead to very detailed maps showing geomorphology and engineering geology features relevant to slope instability.
It will remain very difficult to identify very large, relic and inactive landslides. This exercise has shown that very large, and mostly relic, landslides are often difficult to identify and interpret in the context of Caribbean Islands where a substantial vegetation cover masks topographic features.
This will require much detailed additional geological and geomorphological investigations that falls beyond the current scope of the project.
Digital Elevation Models
The accuracy of the 30 DEMs was derived by comparing the elevation values in the DEMs to the most accurate ancillary elevation data available for that AOI using. For St. Lucia, the ancillary elevation data comprised 18 GPS control points. For Grenada, ancillary elevation data comprised a random sample of 500 LiDAR data points selected independently of those used in the vertical calibration. Accordingly, the vertical accuracy of the DEMs was computed as the root-mean-square (RMS) error:

where ZDEM and Zref are the corresponding DEM and ancillary elevations, respectively, and n is the number of elevation values.
Subsequently, the vertical accuracy of the DEM for St. Lucia was found to be 1.4m, whereas the vertical accuracy for the Grenada DEM was computed as 4.9m. The accuracies of these DEMs therefore exceed the target accuracy of 5–10m.
Service 3: Digital Elevation Models of Belize
National Digital Elevation Model
The 20m DEM provides 40% coverage, and the 30m DEM provides 100% coverage of the Belize DEM; thus exceeding the minimum requirement of 80%. The vertical accuracy (RMS) of the 20m DEM is reported to be in the region of 7m by the data providers. With no other ancillary elevation data available, the 20m DEM was used compute the vertical accuracy of the 30m DEM as in Eq. 3 above. As a result, the 30m DEM was found to have a vertical accuracy (RMS) of 9.8m, which is within the target accuracy of 5–10m.
Precise Digital Elevation Model
As discussed above, the precise 1m DEM for a 100km2 subset of Belize has not yet been produced due to a lack of suitable tri-stereo Pleiades imagery.
Initial Validation
Preliminary data have only recently been released to the ITC project therefore we do not have validation data from the users yet. A workshop/training exercise with the users is planned for end February to early March 2015 and BGS expects to gain more complete feedback from the users at that workshop/exercise.
References
- ↑ Congalton, R. G. (1991). A review of assessing the accuracy of classifications of remotely sensed data. Remote Sensing of Environment, 37, 35–46.
- ↑ Jump up to: 2.0 2.1 Guzzetti, F. (2005). Landslide hazard and risk assessment. Concepts, methods and tools for the detection and mapping of landslides, for landslide susceptibility zonation and hazard assessment, and for landslide risk evaluation (Doctoral dissertation, PhD Thesis, Bonn).
- ↑ Malamud, B. D., Turcotte, D. L., Guzzetti, F., & Reichenbach, P. (2004). Landslide inventories and their statistical properties. Earth Surface Processes and Landforms, 29(6), 687–711.
- ↑ Hurst, M D, Ellis, M A, Royse, K R., Lee, K A and Freeborough, K. (2013). Controls on the magnitude-frequency scaling of an inventory of secular landslides. Earth Surface Dynamics, 1, 67–78.